World-class technologies and expertise at our core facilities and research centers support a multidisciplinary research & innovation ecosystem across Cornell’s campuses.
We support researchers and research administrators through every step of the research endeavor, from proposals to managing projects and ensuring compliance.
Cornell’s offices and programs work with researchers, investors, and industry partners to develop university discoveries for societal benefit.
Research and Innovation at Cornell
Culture of Collaboration
With world-class research facilities and unparalleled quality and breadth of expertise, Cornell fosters a rich environment for collaborations. The complementary resources of Cornell’s rural and urban campuses create unique opportunities to pursue holistic, transdisciplinary approaches to complex challenges.
Academic Integration →
Provost’s Radical Collaboration Initiative →

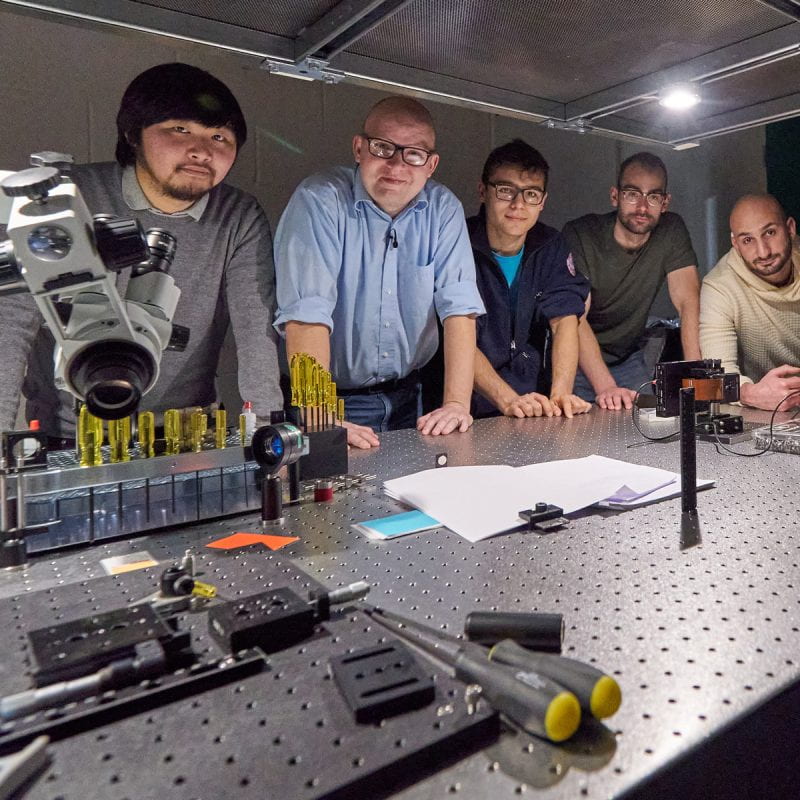
Vibrant Innovation Ecosystem
A network of specialized business incubators, seed funding opportunities, competitions, mentorship programs, and other resources give researchers and entrepreneurs the training and support they need to translate ideas and discoveries into innovations that benefit people and communities around the world.
Innovation and Entrepreneurship Overview →
Center for Life Sciences Ventures →
Center for Regional Economic Advancement (CREA) →
Praxis Center for Venture Development →
Entrepreneurship at Cornell →